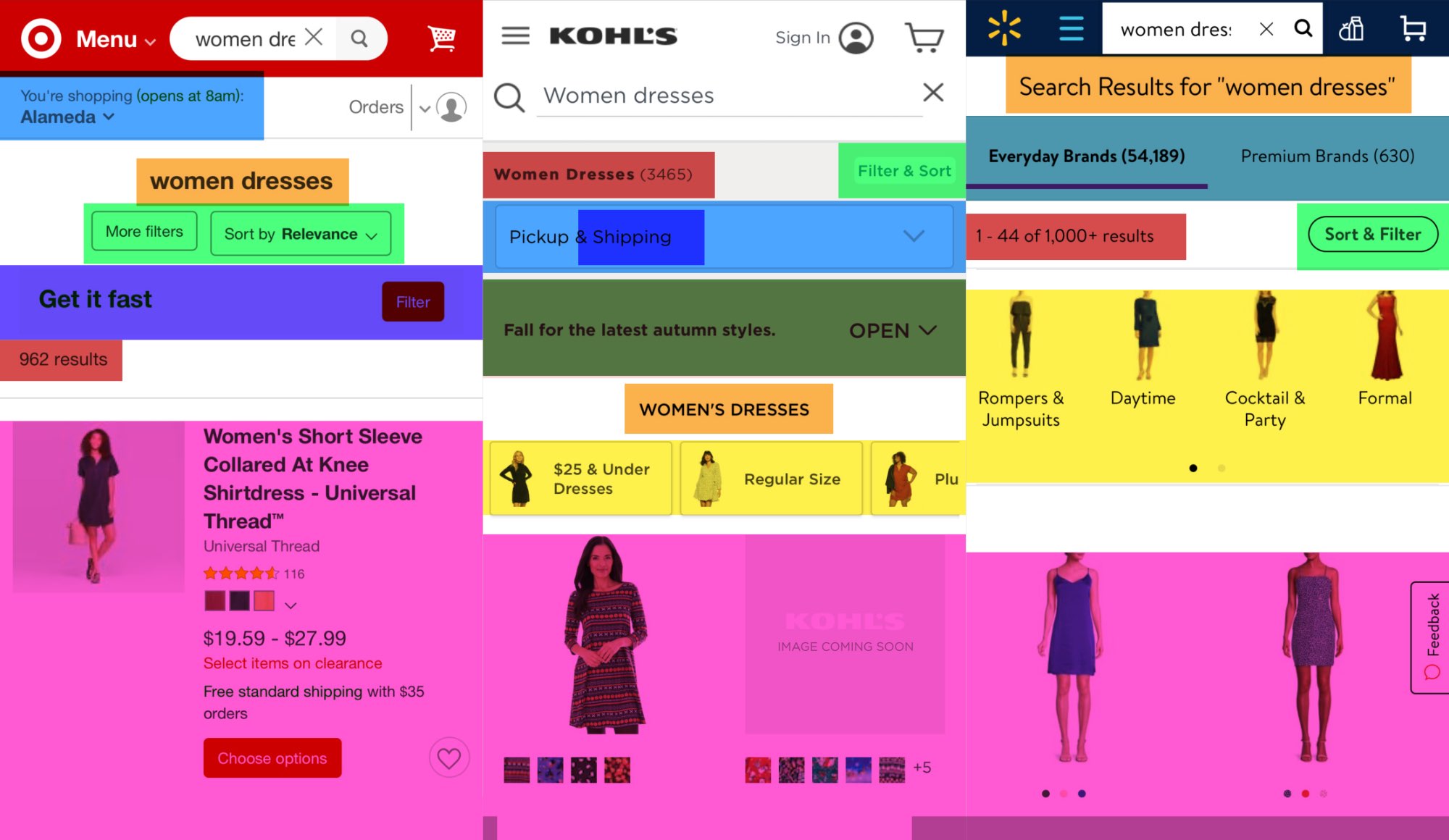
A novel new way to rapidly learn from competitive analysis
Competitive analysis in a business environment constrained for time is typically done more as reconnaissance of feature sets. Time often doesn't afford deep analysis, and drawing inspiration usually comes from biased opinions, a shiny object, basic heuristics, or perceived trends.
At Macy's, we developed an interesting new process where we could rapidly gain deep insights without too much effort and safeguarded against individual bias or opinions.
Our user's problem
Our approach was tested with a simple business problem. Users browsing our pages on mobile barely saw any product on page load, a result of disparate features bloating the top of the pages.
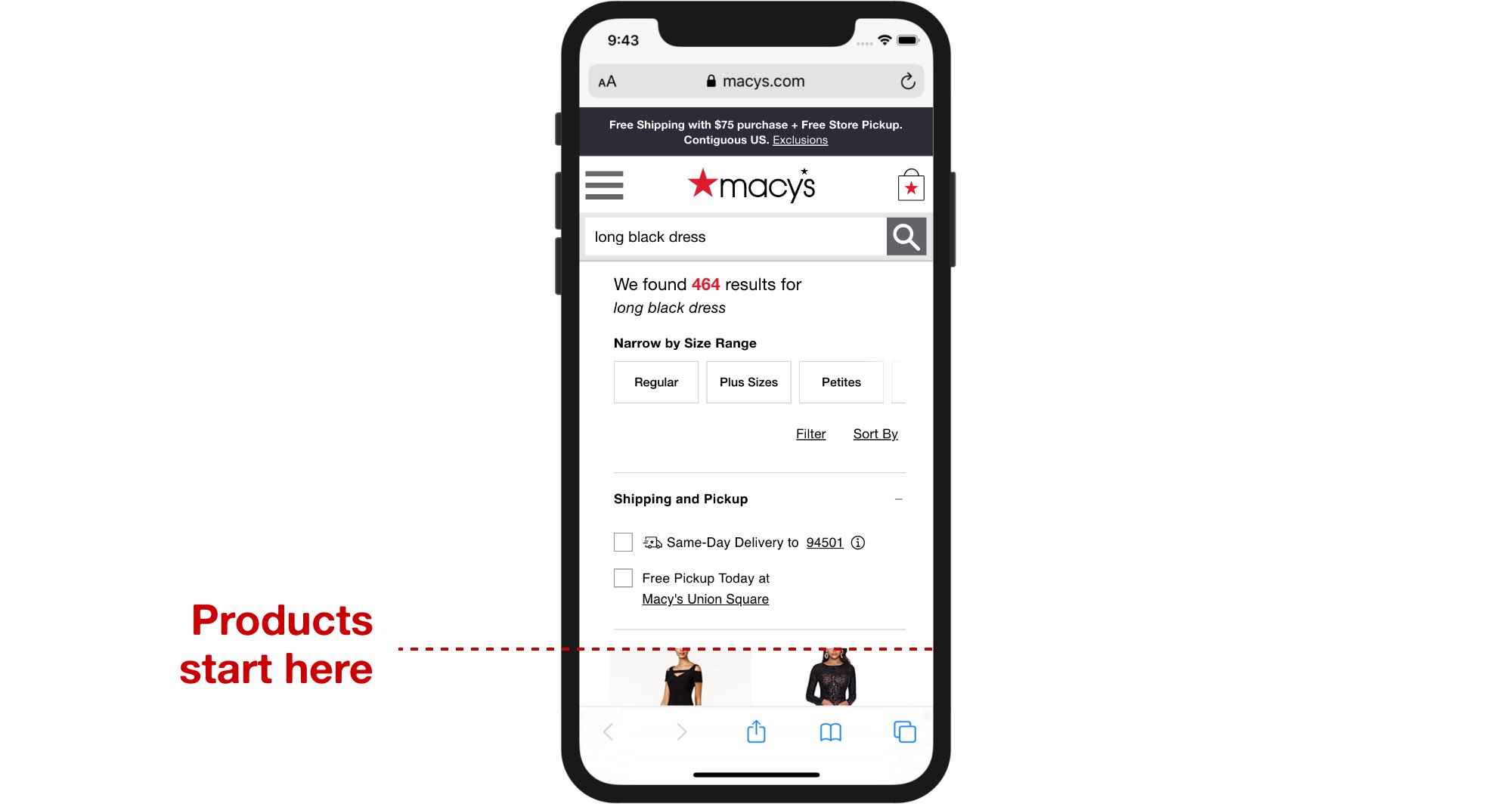
A look at our competitors
As we looked at competitors, we compared the size, position, and overall priority of each feature. This helped to draw a holistic view of how competitors were doing better. But at the individual feature level, the debate typically turned to differing opinions. But at the end of the day, we knew only user tests or experimentation would reveal more true assertions.
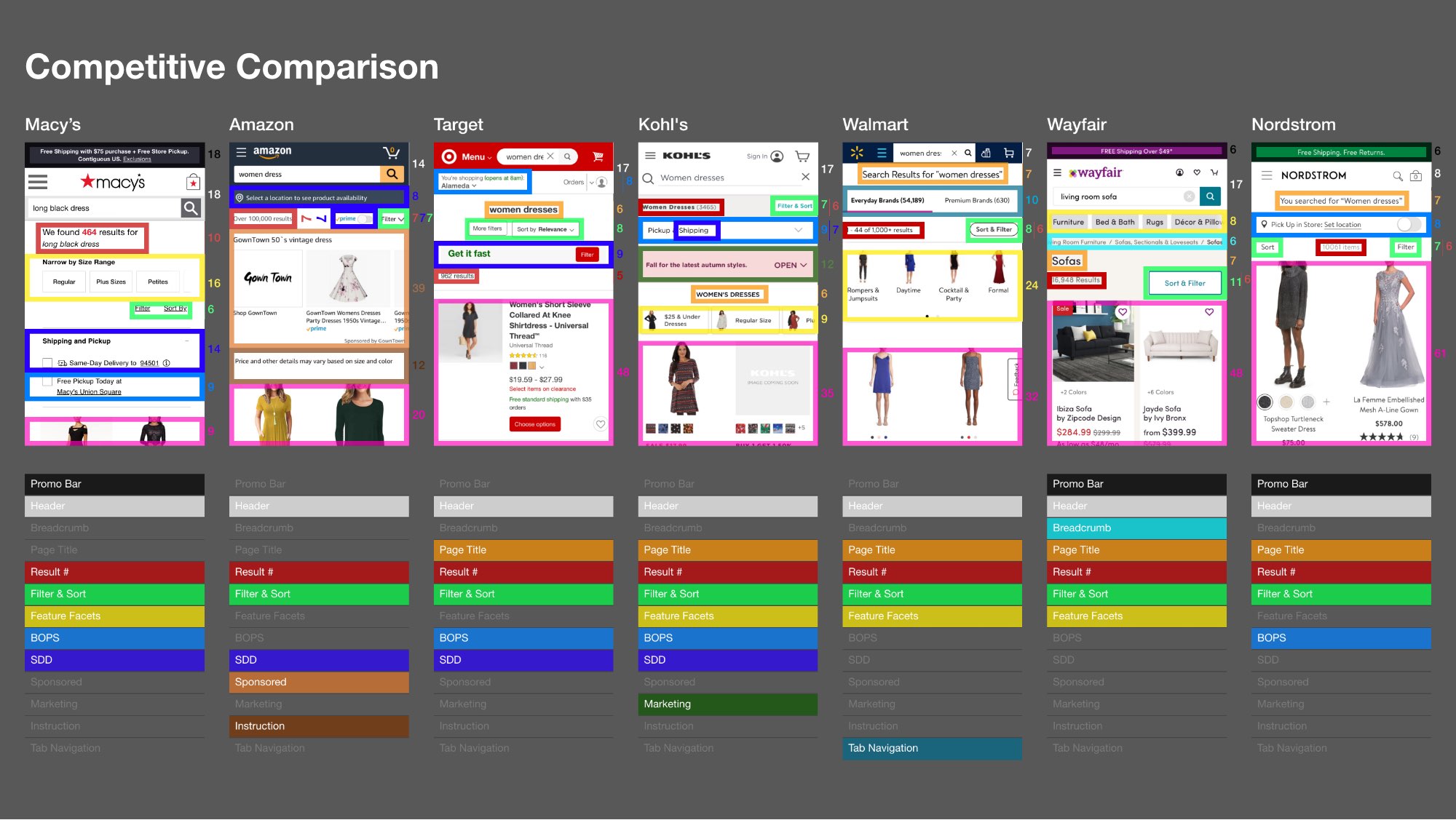
What if we just copied and tested?
You know, like when you just copy the answers from the classmate sitting next to you. It was kind of like that, but for design.
This was not intended as final design recommendations, but more as a fast brute force method to simply copy our competitors dressed up in Macy's style and test them against quick user tasks. The designs were rough and intentionally throwaway, our goal being quick insights. Replication also had the effect of putting each interface on equal stylistic footing.
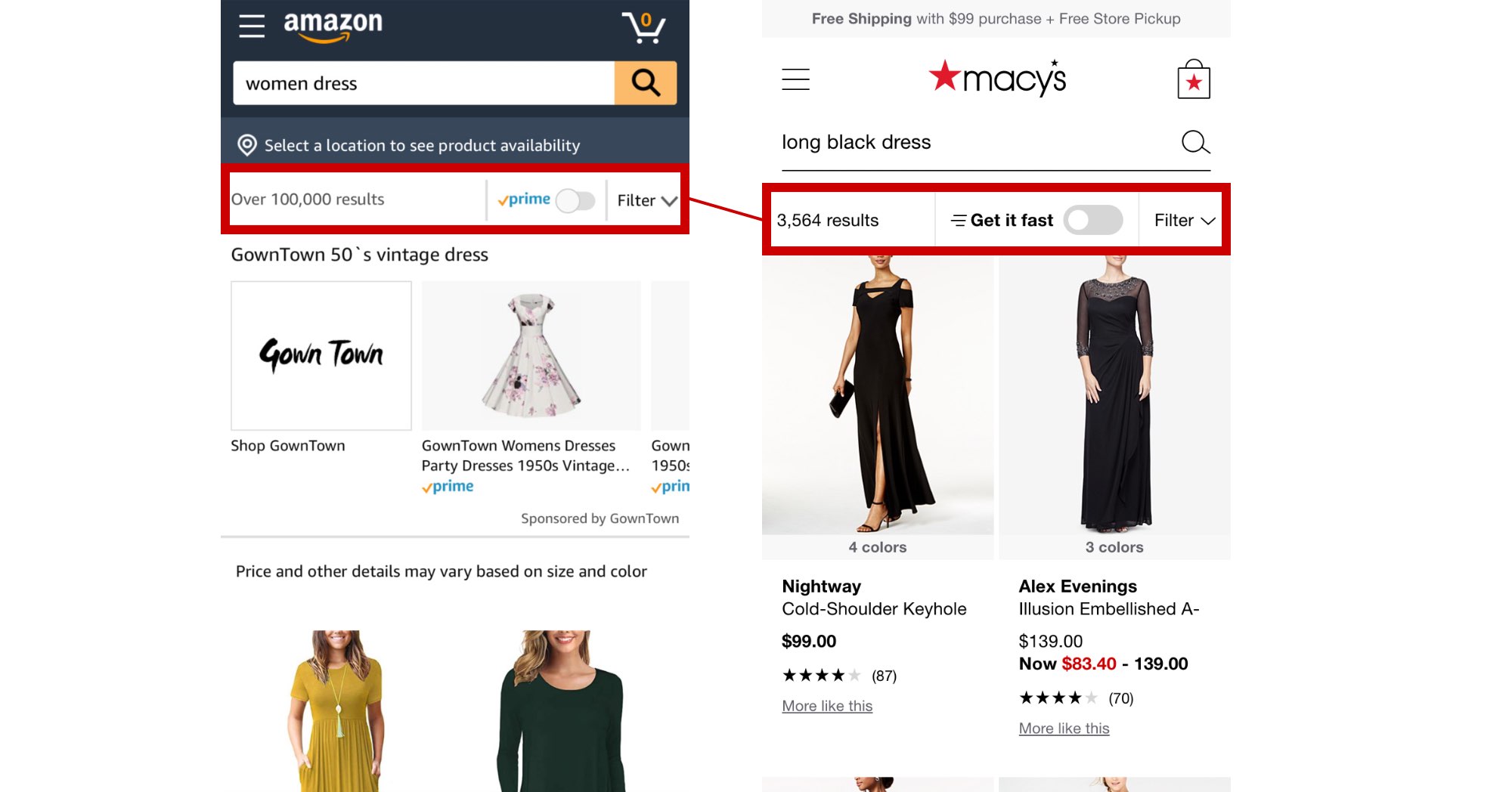
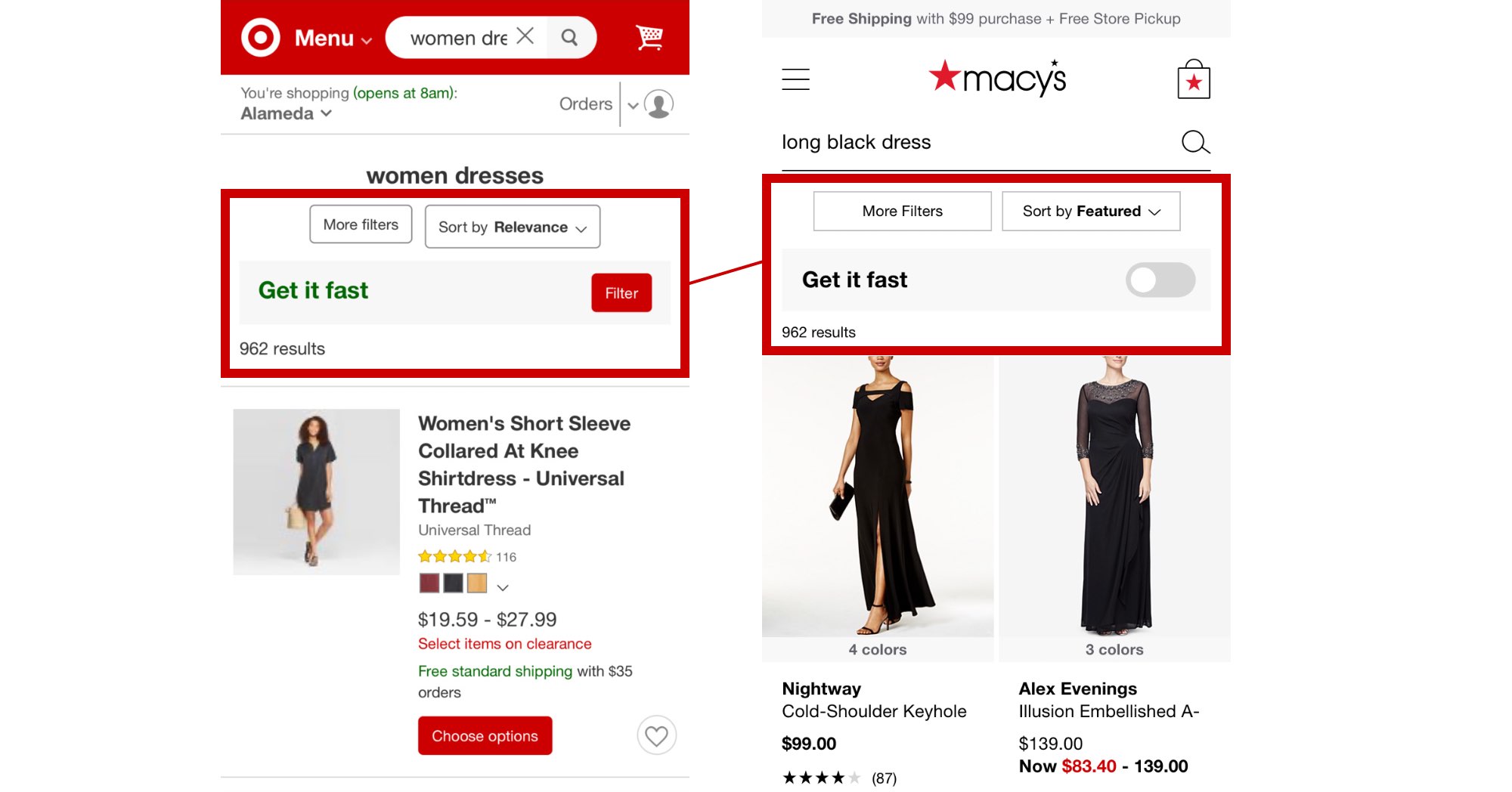
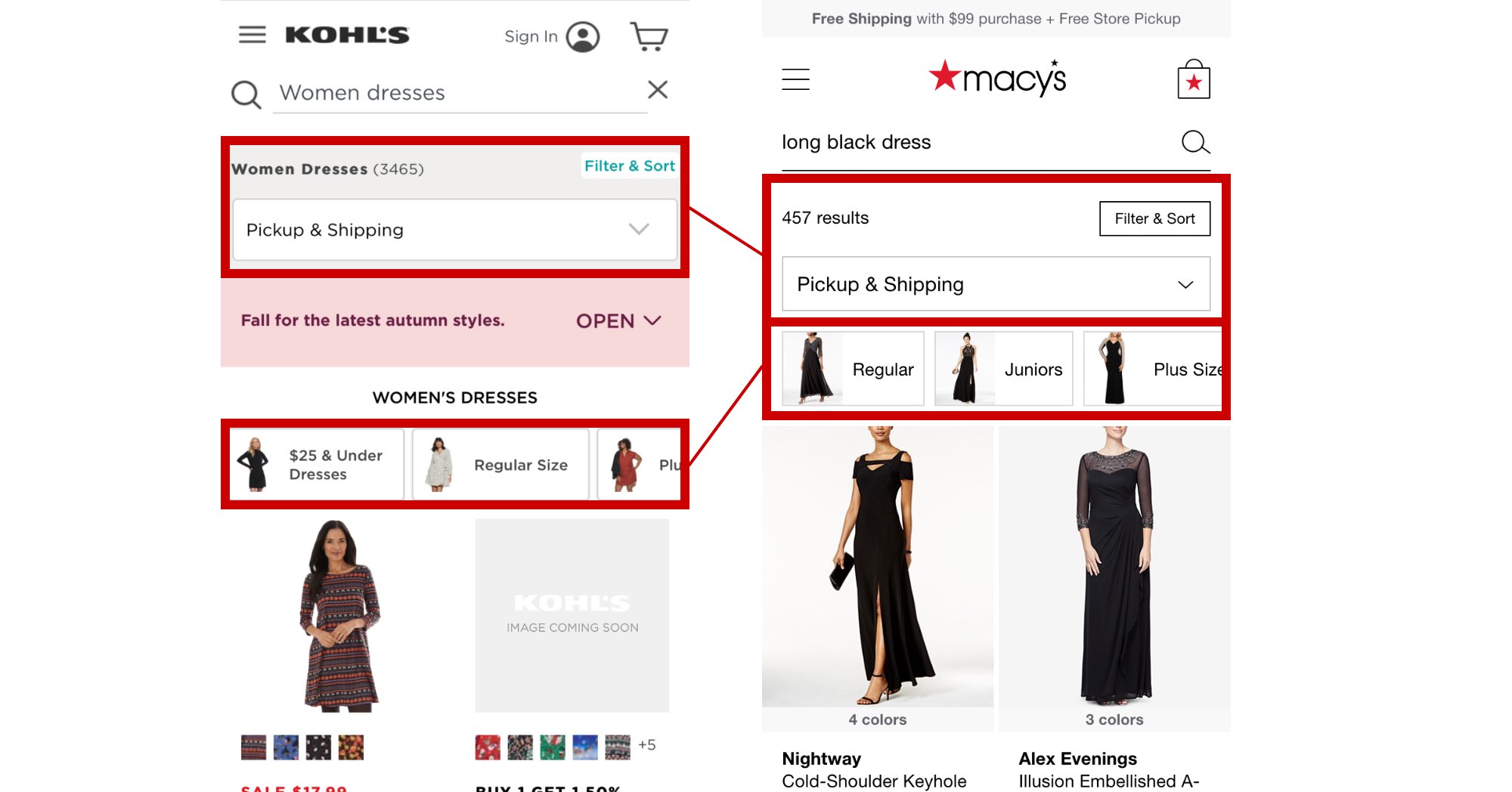
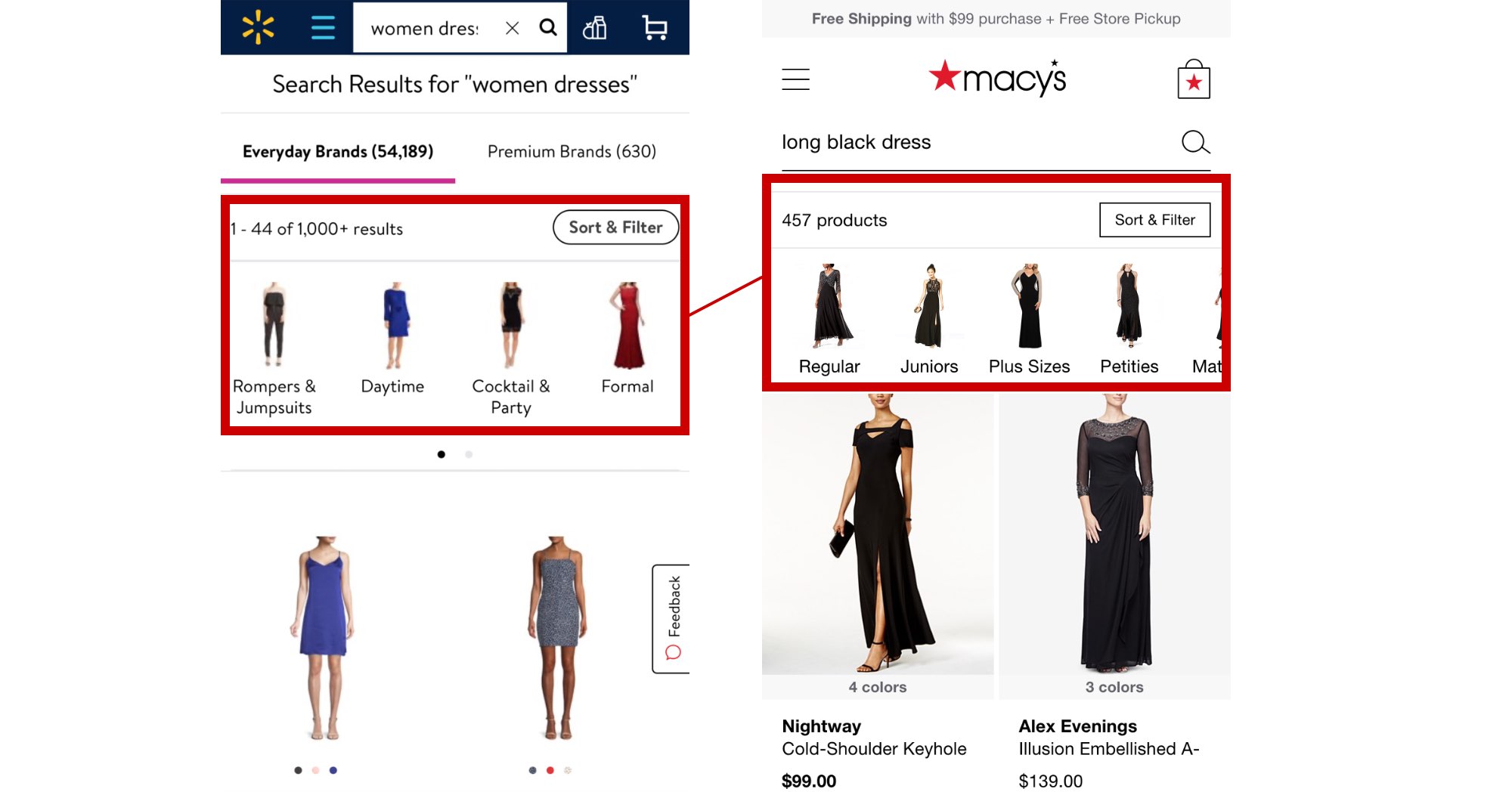
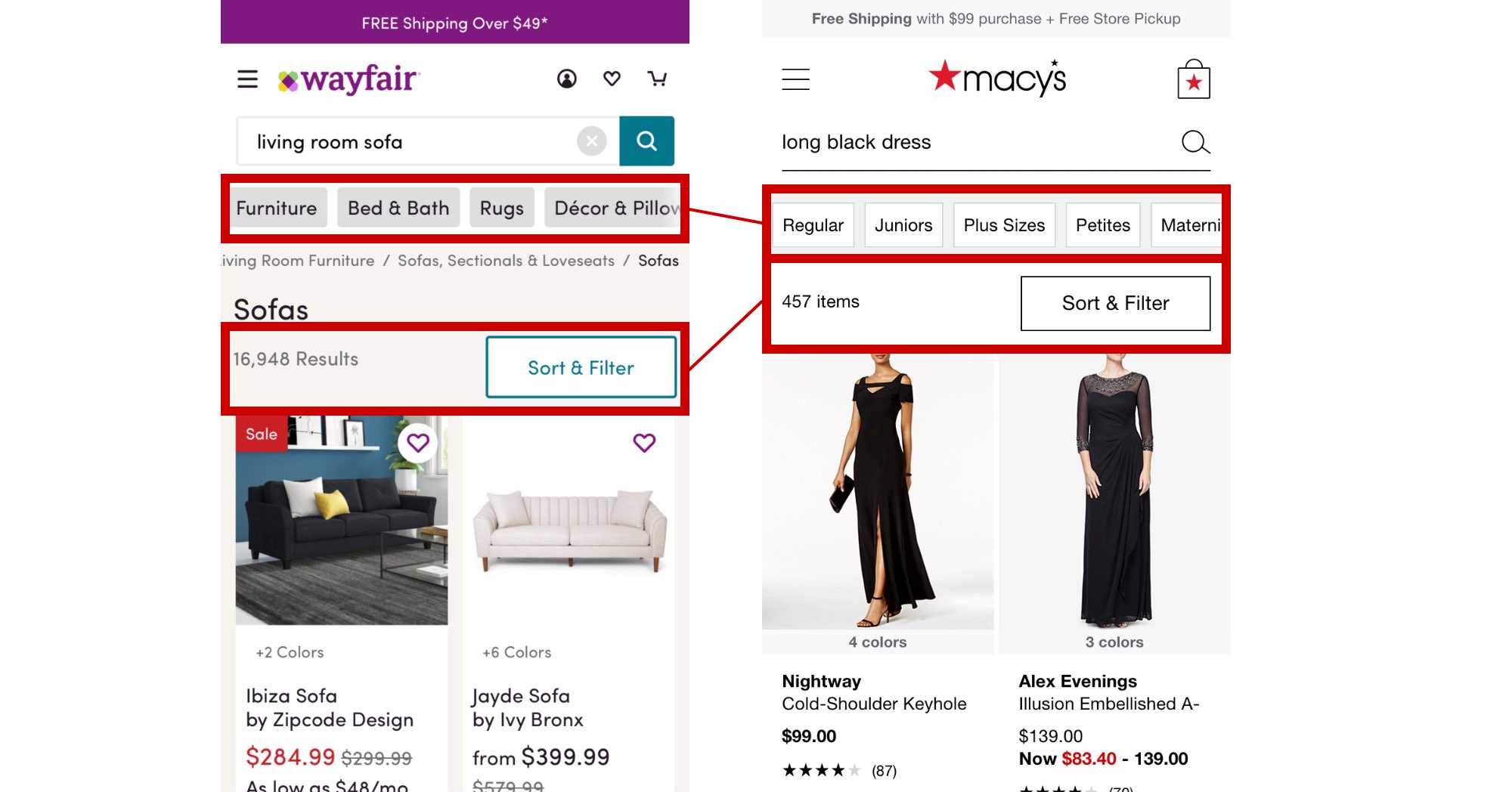
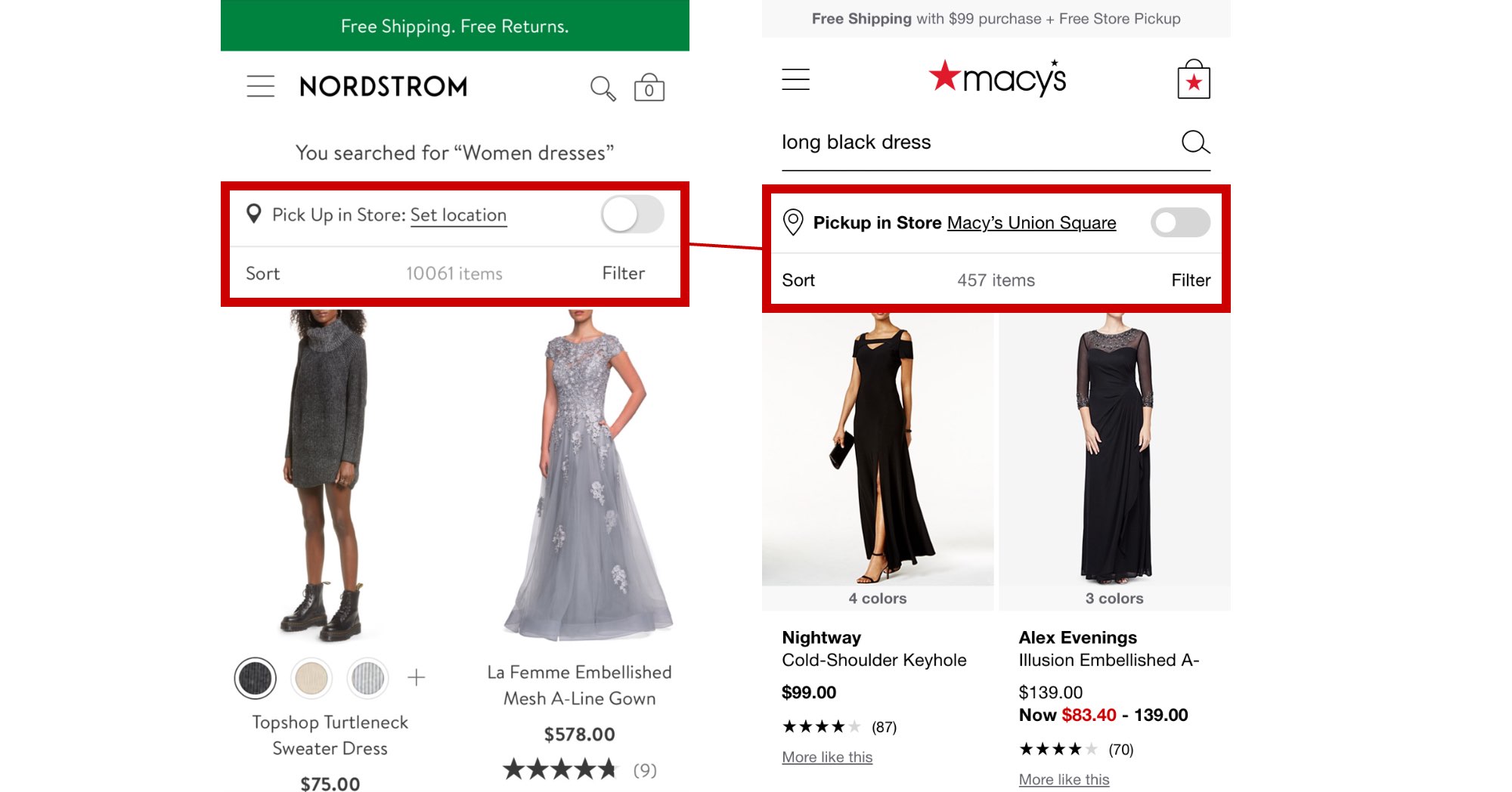
Including our own experiments and control
We also added our own concepts plus the current control in user testing to see how they would fare directly against the replicants.
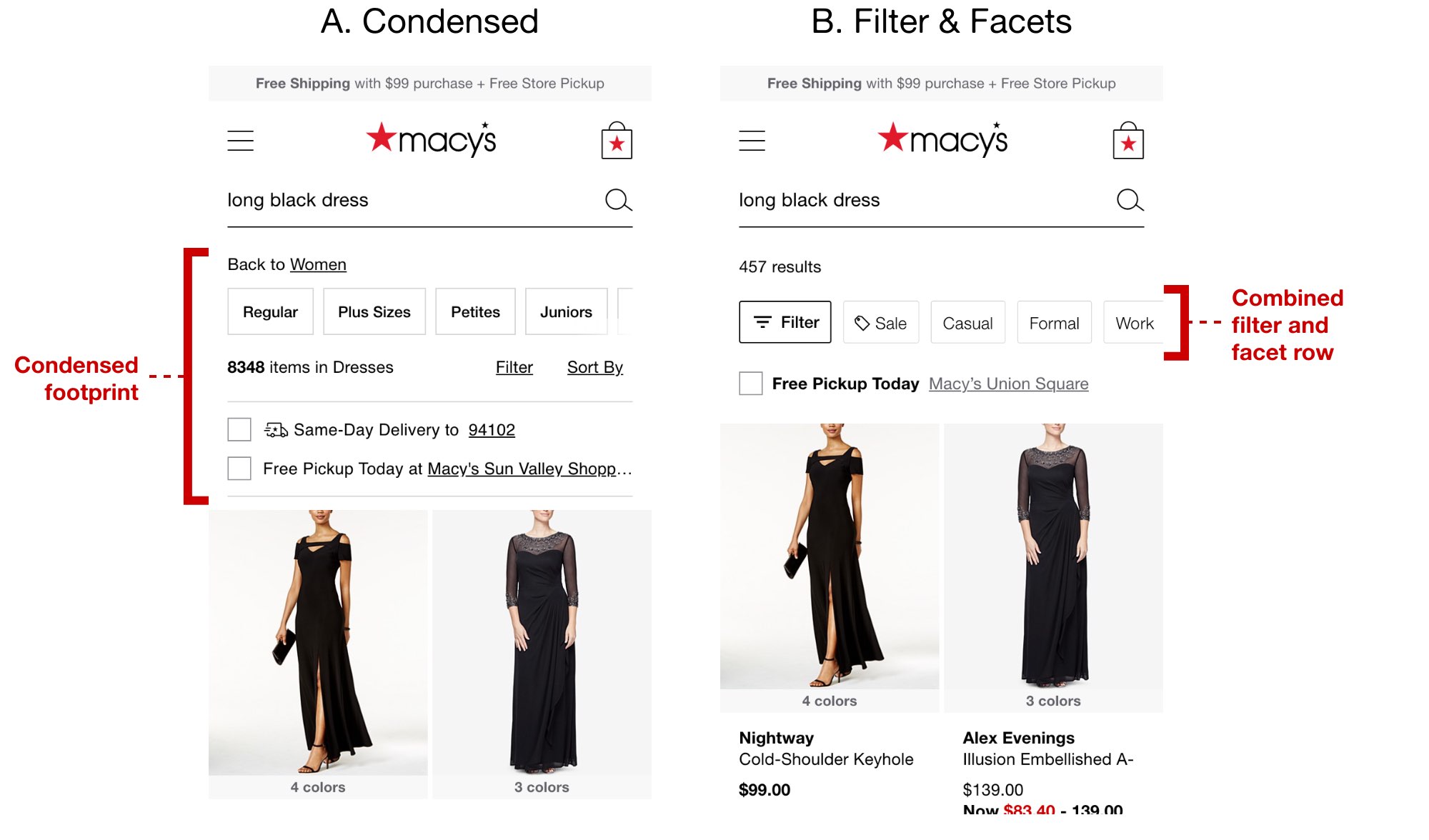
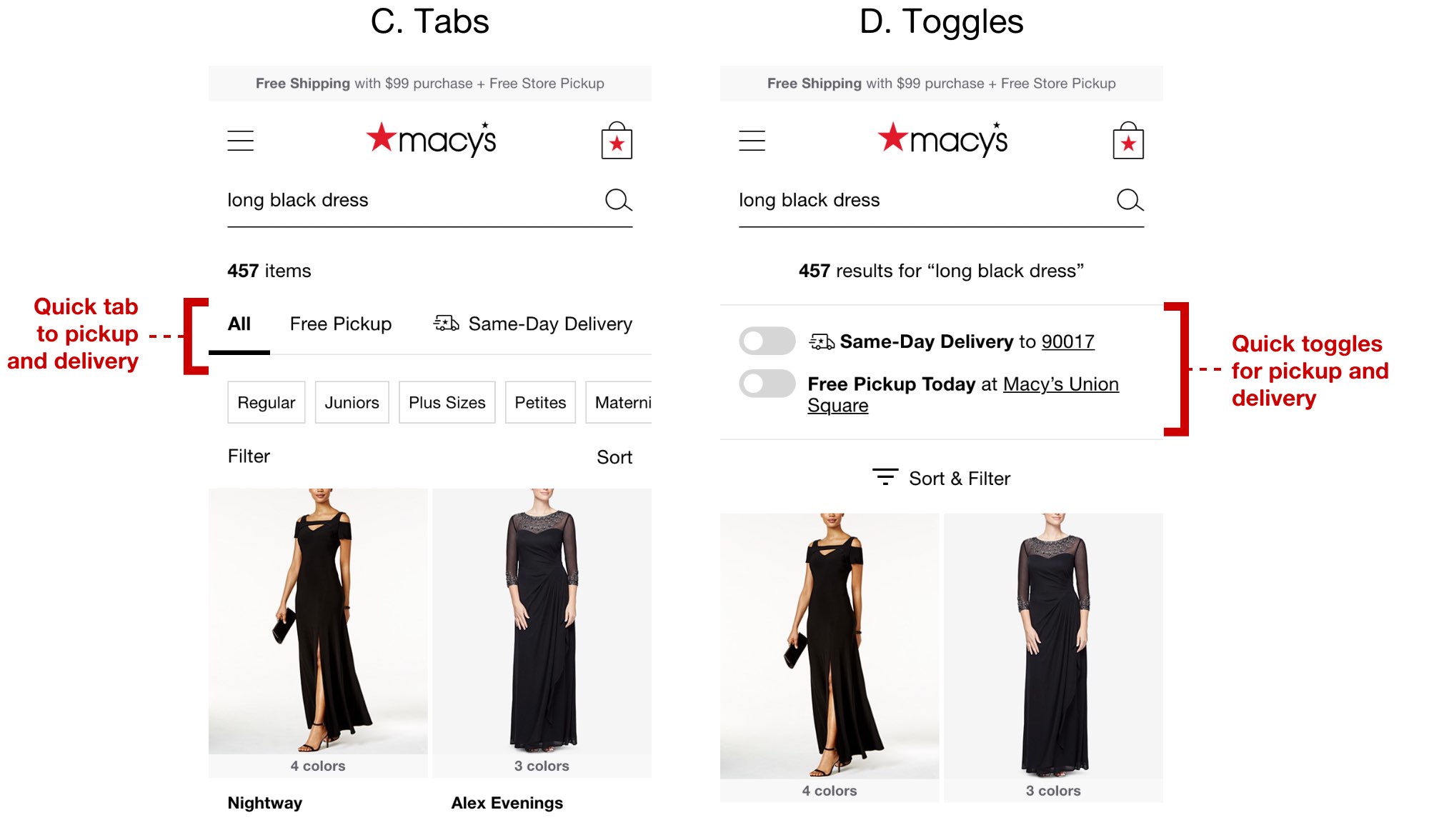
Usability results
Prior to the results being revealed, we polled the team on which they thought would be the winner. Opinions again were largely based on heuristics with perceived applicability to our business and perceptions on how users would likely respond.
Of over 30 people, only one guessed the winning treatment: the Amazon replicant.
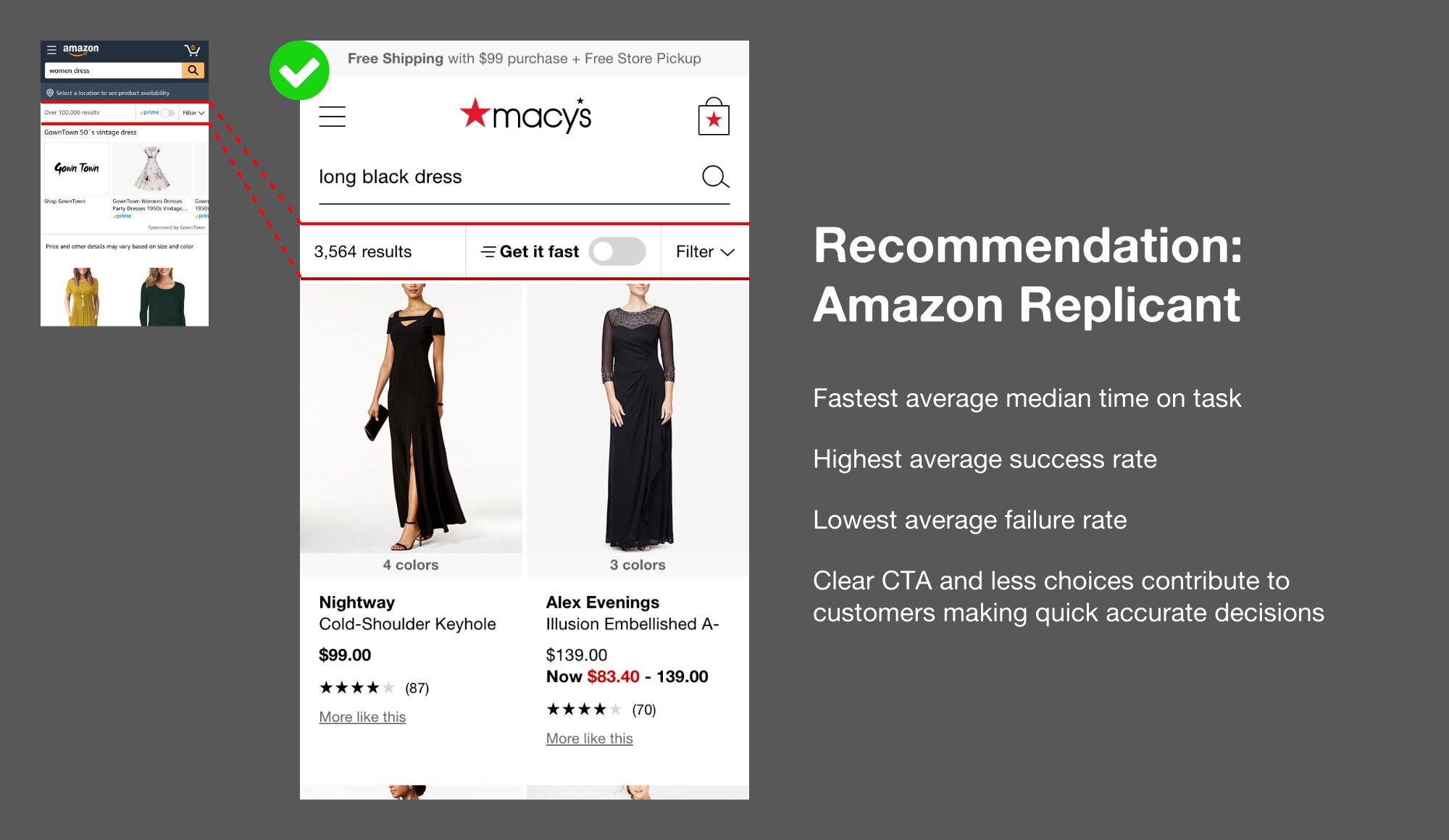
Iteration and expansion for experimentation
This was a big eye opener to the team, providing self-realization to all, myself included, to be careful of our design biases, to be more intentional in challenging our own beliefs. We continue to mature this process for future projects, likely applicable for more simple and discrete features that are easily replicated with little fuss.
We made new iterations inspired by the Amazon replicant currently in development for experimentation.